Blogs
How intelligent? What the age of AI means for your organization
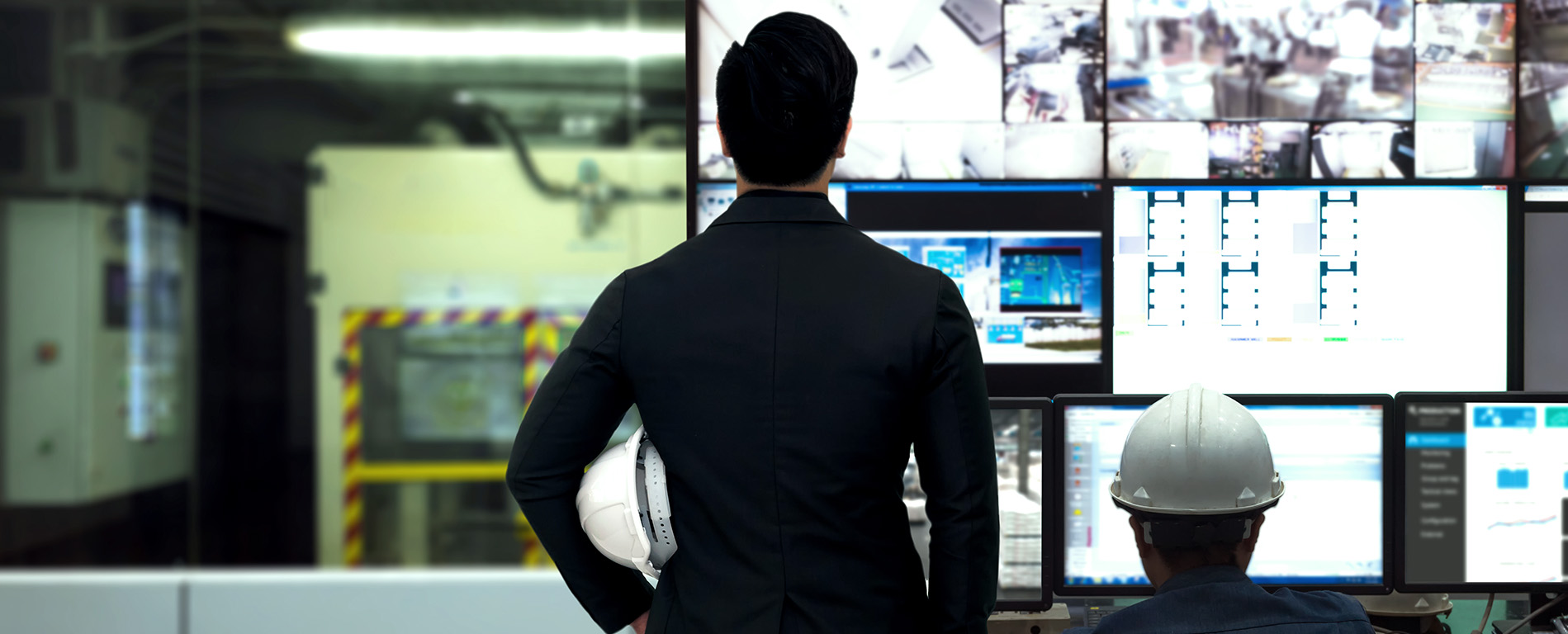
Mention the term AI, and you will witness a dizzying variety of thoughts and emotions. From imagination to fear, from excitement to paranoia, it invokes either a sense that the opportunities are endless or that the world is coming to an end. For some it is heaven, for others it is hell. AI provokes reactions as numerous as its applications.
AI is also the third of the four most-discussed topics at Gartner’s 2018 Conference and IT Expo. In this article, I’ll take you on a whirlwind tour through AI in today’s market: its recent history, current applications, and ethical complexities.
History
The term AI was coined in 1956 in a historic conference at Dartmouth College, but the belief in artificial beings endowed with intelligence existed long before. The famous prediction, “Within one generation the problem of creating ‘artificial intelligence’ will substantially be solved” sparked imaginations across the globe. However, like predictions of rock and roll’s demise, high-speed travel, or “fads” such as the automobile, television, or smartphone, it was quite inaccurate.
So great was the disillusionment, that for most of three decades between the 70s and 90s, experts lamented an ‘AI winter,’ a barren stretch in which funding and exploration lay silent. The promise of AI was relegated to sci-fi movies.
You may remember the springtime of the late 90s and early 2000s. IBM’s Deep Blue became the first computer to beat a chess champion when it defeated Russian grandmaster Garry Kasparov. IBM’s Watson won the quiz show Jeopardy over reigning human champions Brad Rutter and Ken Jennings.
Despite this AI renaissance, skeptics were unconvinced. Many openly doubted that computer processing power could ever match human capabilities. Others contended that the issue wasn’t processing power but streams: that there was simply not enough data for machine learning to reach adequate maturity.
In recent years, the rise of Big Data has settled these doubts. The data available to machines has far surpassed necessary thresholds. With billions of parameters, we can now train computers to recognize incredibly subtle patterns within mountains of data—exponentially more data than a human brain could even begin to process. This explosion of data has catapulted AI from the realm of imagination to that of anticipation.
Yet resolving one concern has raised another. In the age of Big Data, organizations have more data points than they could ever actually use. Today’s challenge is no longer about capturing and storing data. The chief difficulty, for both AI and the organizations that will use it, lies in filtering data, in framing and arranging information for optimal decision-making.
Misconceptions
We tend to view Artificial intelligence as autonomous computing that meets and exceeds human capabilities. This perspective is misleading. In reality, intelligence is never artificial. Intelligence comprises multiple factors, from creativity and curiosity to critical thinking. Whether machine learning or chatbots, the goal of AI is not to make processing independent or even to perform it faster. It is to augment people with intelligent systems that maximize both efficiency and effectiveness.
Consider the following statistics…
AI/ML spending is expected to increase from ~$14B in 2018 to ~$58B by 2021.
AI jobs have increased 450% since 2013.
61% of organizations identified ML/AI as their company’s most significant data initiative for 2019.
70% of organizations say that they have limited or no skills or understanding in the realm of AI technologies.
Why do organizations pursue AI? The most common reasons include agility, improved customer experience, cost savings due to automation, and increased revenue. Secondary reasons include the first mover advantage, better employee engagement, customer demand, market disruption, and a fear of falling behind.
The promises of AI are both now and not yet. Today’s AI-enabled organizations can reasonably expect enhanced decision-making, process automation, and advanced VPAs. Yet some advantages remain theoretical. Organizations should expect these investments to generate little to no ROI until 2025 and beyond: self-learning robots, embedded AI products that learn and adapt to owners, and cognitive analytics.
The speakers at Gartner’s IT Expo gave a sobering view of AI realities. 86% of innovative technologies surrounding AI will die in the trough of disillusionment within the next two years. 54% of technologies that succeed are expected to plateau until after 2022. One speaker estimated that 41% of successful technologies will fall short of transformative impact until 2027.
Application
When considering what AI applications make sense for your business, consider how other industries have implemented it. For example, conversational AI for hands-free operation in manufacturing can also bring value in retail and healthcare. Principles driving predictive diagnosis in healthcare apply to transportation and IT services.
Properly applied, machine learning can solve both common and complex business problems. Judging by the Gartner presentations, I would identify the three most common AI strategies as:
- (Most popular) Making predictions based upon learned patterns from historical data.
- Organizing and simplifying data, then making predictions based upon inference.
- Learning by trial and error. The algorithm prescribes a solution, gauges consequences (good or bad), and adjusts its outputs to enhance positive and reverse negative outcomes.
An effective AI strategy will provide or enable the following functionalities:
- Insights: predictive analytics, patterns and trends, modeling, forecasting, personalization
- User experience: NLP, specialized devices, language translation, voice recognition, robotic or conversational assistants; secure, increase engagement, service enhancement, ease of use
- Process improvement: IoT, robotics, AI imbedded business applications; optimization of assets and operations, service enhancement, resource conservation, grow revenue, reduce cost
- Security: applicant screening, investment research, cybersecurity, fraud detection/prevention
Yes, the possibilities do seem limitless. But there are limitations. For example, AI only functions within clearly defined domains. AIs cannot simulate generalized intelligence or common sense. An AI designed to beat human chess or Go champion cannot either self-reflect or play other games. Robots that can assemble a car cannot assess the likelihood of loan default.
Prior to any AI implementation, ask yourself whether the intended benefit is worthwhile. Peter Drucker laments the number of organizations that fall into a common trap: “There is nothing so useless as doing efficiently that which should not be done at all.”
Build structure into every AI implementation. You should begin with a clear and thorough understanding of your organization’s context and business drivers. This understanding should then inform a hypothesis, or a series of hypotheses. Each stage of the implementation should be carefully tested and tweaked accordingly.
The Ethical Question
The best of AI works with human nature rather than against it. AI cannot replace authentic human wisdom and intelligence. It cannot substitute for ordinary observance, unfettered optimism, gut feel, common sense, and spontaneous emotion. But it can offset human shortcomings.
Take our innate unpredictability. The same stimulus rarely produces the same response twice. Even with rigid criteria, humans fail to produce consistent results. Each hour, Humans improperly sort about 18,400 pieces of mail and deduct 22,000 checks from the wrong bank accounts. Each year, we mismatch 114,500 pairs of shoes and print 2,500,000 books with incorrect covers. AI allows us to achieve and maintain a level of consistency manual labor could never dream of.
However, “Predicting what technology will do is much easier than predicting what humans will do with it.” Craig Roth’s quip could not be more apt. No technology admits an unequivocal judgment of good or bad, empowering or dangerous. AI is not a set of propositions. It is an immensely powerful force of almost universal applicability. It can reinforce dictatorships as well as solve famines. As such, AI requires clear and unequivocal guidelines.
Implementing AI of any sort thrusts ethical responsibility upon your organization. Every adopter will need to thoroughly consider their organization’s response to a range of ethical issues. How will you go about firing employees whose jobs have been automated? What safeguards will you put in place to mitigate artificial stupidity and unintended consequences? Do you know the full extent of your algorithm’s selection bias? Would you trust your own information to the adaptive system you’ve created for employee records?
AI suggests autonomy, but artificial intelligence is anything but. Human critical thought guides AI processing. We must maintain a reflective and independent mindset. We must carefully construct and evaluate arguments, understand logical connections, reason our way through inconsistencies and mistakes, systematize problem-solving approaches, and challenge the conclusions of others as well as ourselves. Only as we grow and develop our own intellects can we effectively code machines for responsible thought and reasoning.